

In this research, the authors propose a predictive analytics framework for crane configuration selection using combined heuristic search and artificial neural network (ANN) approaches for heavy industrial construction projects. In the planning process of heavy industrial construction projects, mobile crane selection plays a critical role in the project’s success, and poor choice of mobile crane configurations can lead to unnecessary cost-overrun and delayed schedules.

Predictive analytics have been used to improve efficiency and productivity in the construction industry by leveraging the insights from historical data with a variety of applications in project management. At last, a case is represented in order to demonstrate the use of the developed algorithm and to illustrate its essential features. The algorithm is incorporated into a three-dimensional (3D) computer-aided system that integrates crane selection module, crane modeling module, 3D-simulation module, 3D computer-aided design modeling module, rigging calculation module, and data management module. This paper presents a newly developed algorithm for selecting mobile cranes on construction sites, which takes into account the lifting capacity, the geometrical characteristics of the crane, the dimensions of equipments and riggings, and the ground bearing pressure. It is a tedious job that lifting planners select cranes for construction projects based on a large number of lifting capacity charts. These charts are structured based on predetermined crane configurations, which consist of boom/jib length, lifting radius, main boom angle to ground, and jib angle to ground or its offset to its main boom centerline.
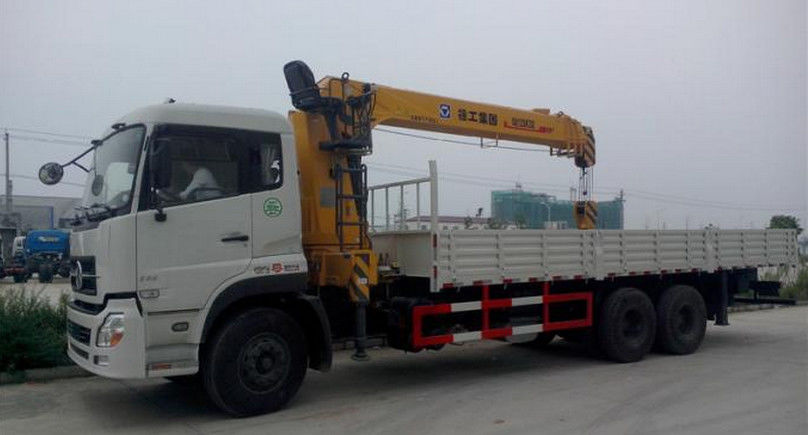
Lifting capacity charts are tabulated and provided to operators and practitioners by mobile crane manufacturers.
